Expert Machine Learning and Deep Learning Techniques for Developing Smarter IoT

The Internet of Things (IoT) is a rapidly growing field that is having a major impact on the way we live and work. IoT devices are already being used in a wide variety of applications, from home automation to industrial manufacturing. As the number of IoT devices continues to grow, so does the need for more intelligent and autonomous systems.
4 out of 5
Language | : | English |
File size | : | 23953 KB |
Text-to-Speech | : | Enabled |
Screen Reader | : | Supported |
Enhanced typesetting | : | Enabled |
Print length | : | 392 pages |
Machine learning and deep learning are two essential techniques for developing smarter IoT devices. Machine learning algorithms can be used to learn from data and make predictions, while deep learning algorithms can be used to learn complex patterns from data. These techniques can be used to create IoT devices that are more intelligent, efficient, and responsive.
Machine Learning Techniques for IoT
There are a wide variety of machine learning techniques that can be used for IoT applications. Some of the most common techniques include:
- Supervised learning: Supervised learning algorithms learn from labeled data, which means that the data is already associated with the correct output. This type of learning is used to train models that can predict the output of new data, such as the temperature of a room based on the current temperature and humidity.
- Unsupervised learning: Unsupervised learning algorithms learn from unlabeled data, which means that the data is not associated with the correct output. This type of learning is used to find patterns in data, such as the groups of customers who are most likely to buy a particular product.
- Reinforcement learning: Reinforcement learning algorithms learn by interacting with their environment and receiving feedback. This type of learning is used to train models that can make decisions, such as the best route to take to a destination.
Deep Learning Techniques for IoT
Deep learning is a type of machine learning that uses artificial neural networks to learn from data. Neural networks are inspired by the human brain and can learn complex patterns from data. This makes them ideal for tasks such as image recognition, natural language processing, and speech recognition.
Some of the most common deep learning techniques used for IoT applications include:
- Convolutional neural networks (CNNs): CNNs are used for image recognition tasks. They can learn to identify objects in images, even if the objects are occluded or in different positions.
- Recurrent neural networks (RNNs): RNNs are used for natural language processing tasks. They can learn to understand the meaning of text, even if the text is long or complex.
- Autoencoders: Autoencoders are used for unsupervised learning tasks. They can learn to reconstruct input data, which can be used to find patterns in data.
Applications of Machine Learning and Deep Learning in IoT
Machine learning and deep learning techniques can be used to develop a wide variety of IoT applications. Some of the most common applications include:
- Predictive maintenance: Machine learning algorithms can be used to predict when IoT devices are likely to fail. This information can be used to schedule maintenance before the device fails, which can help to prevent downtime and improve efficiency.
- Quality control: Machine learning algorithms can be used to inspect products for defects. This can help to ensure that only high-quality products are shipped to customers, which can improve customer satisfaction and reduce costs.
- Energy management: Machine learning algorithms can be used to optimize energy usage in IoT devices. This can help to reduce energy costs and improve sustainability.
- Security: Machine learning algorithms can be used to detect security threats in IoT devices. This can help to protect devices from unauthorized access and data breaches.
Machine learning and deep learning are essential techniques for developing smarter IoT devices. These techniques can be used to create IoT devices that are more intelligent, efficient, and responsive. This has the potential to revolutionize the way we live and work, and to create a more sustainable and connected world.
4 out of 5
Language | : | English |
File size | : | 23953 KB |
Text-to-Speech | : | Enabled |
Screen Reader | : | Supported |
Enhanced typesetting | : | Enabled |
Print length | : | 392 pages |
Do you want to contribute by writing guest posts on this blog?
Please contact us and send us a resume of previous articles that you have written.
Book
Novel
Story
Library
Paperback
E-book
Sentence
Glossary
Bibliography
Preface
Synopsis
Footnote
Tome
Bestseller
Library card
Narrative
Biography
Encyclopedia
Thesaurus
Narrator
Resolution
Librarian
Catalog
Card Catalog
Archives
Periodicals
Study
Research
Scholarly
Academic
Journals
Reading Room
Rare Books
Study Group
Dissertation
Storytelling
Awards
Reading List
Book Club
Theory
Amit Rachman
Trina Howell
Robert Young
Suellen May
Dick Blankenship
Raj Athwal
Lisa Jenn Bigelow
Shelle Hendrix
Pia Silvani
Ken Wilkerson
Paul Vickory
Masashi Rotte
Pilgrim David
John Clayton Thomas
Penny Carlile
Samuel P Huntington
Ryan Peek
Graeme Thomson
James Dashner
Jim O Neil
Light bulbAdvertise smarter! Our strategic ad space ensures maximum exposure. Reserve your spot today!
- Duane KellyFollow ·17.8k
- Sean TurnerFollow ·8.9k
- Devin CoxFollow ·18.1k
- Donald WardFollow ·7.5k
- Dave SimmonsFollow ·14.4k
- Devon MitchellFollow ·16.9k
- James GrayFollow ·6.2k
- Junichiro TanizakiFollow ·2k
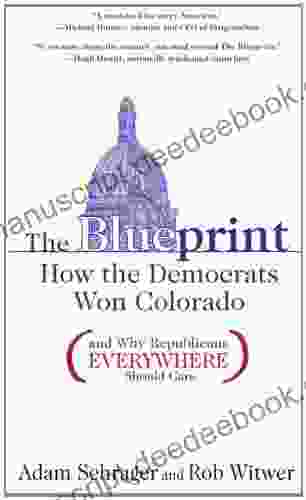

How The Democrats Won Colorado And Why Republicans...
The Democrats' victory...
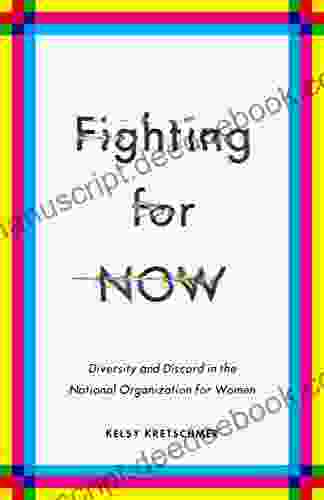

Global Responses to Human Security Threats: Global...
Human security...
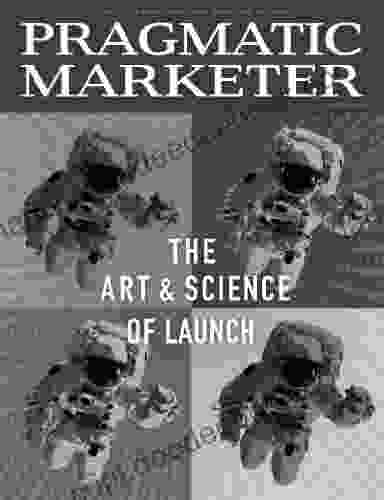

The Product Management and Marketing Authority: Unlocking...
In today's competitive business landscape,...
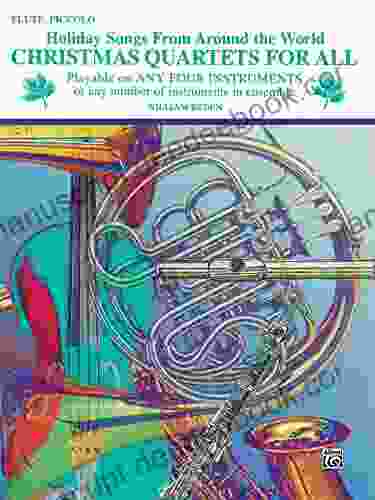

Christmas Quartets For All: A Choral Celebration of the...
Christmas is a time for family, friends,...
4 out of 5
Language | : | English |
File size | : | 23953 KB |
Text-to-Speech | : | Enabled |
Screen Reader | : | Supported |
Enhanced typesetting | : | Enabled |
Print length | : | 392 pages |